Bi rads 4的問題,我們搜遍了碩博士論文和台灣出版的書籍,推薦羅崇銘寫的 人工智慧與影像知識詮釋化(修訂版) 和羅崇銘的 人工智慧與影像知識詮釋化都 可以從中找到所需的評價。
另外網站乳房攝影的解讀系統,BI-RADS也說明:乳房攝影的解讀系統,BI-RADS · 1:正常。 · 2:良性發現。 · 3:可能為良性發現。 · 4:懷疑異常。 · 5:高度懷疑為惡性腫瘤。 · 6:切片已證實為惡性腫瘤。
這兩本書分別來自元華文創股份有限公司 和元華文創股份有限公司所出版 。
國立清華大學 生醫工程與環境科學系 彭旭霞所指導 賴志慶的 前列腺癌核磁共振影像的直方圖分析與深度卷積神經網絡 (2021),提出Bi rads 4關鍵因素是什麼,來自於前列腺癌、直方圖分析、核磁共振影像、深度卷積神經網絡、影像分割、動態對比增強影像、多參數核磁共振影像、擴散加權影像、T2加權影像。
而第二篇論文臺北醫學大學 國際醫學研究碩士學位學程 陳榮邦所指導 VU PHAM THAO VY的 Machine learning algorithm for classification of Ductal carcinoma in situ and minimal invasive breast cancer (2021),提出因為有 Ductal carcinoma in situ (DCIS)、minimal invasive breast cancer、machine learning、ultrasound imaging、mammographic imaging的重點而找出了 Bi rads 4的解答。
最後網站Evaluation of the positive predictive value (PPV3) of ACR ...則補充:BI-RADS category 5 is reserved for cancer-like lesions on diagnostic imaging. BI-RADS 4 includes suspicious lesions with some likelihood of malignancy [3,4].
人工智慧與影像知識詮釋化(修訂版)
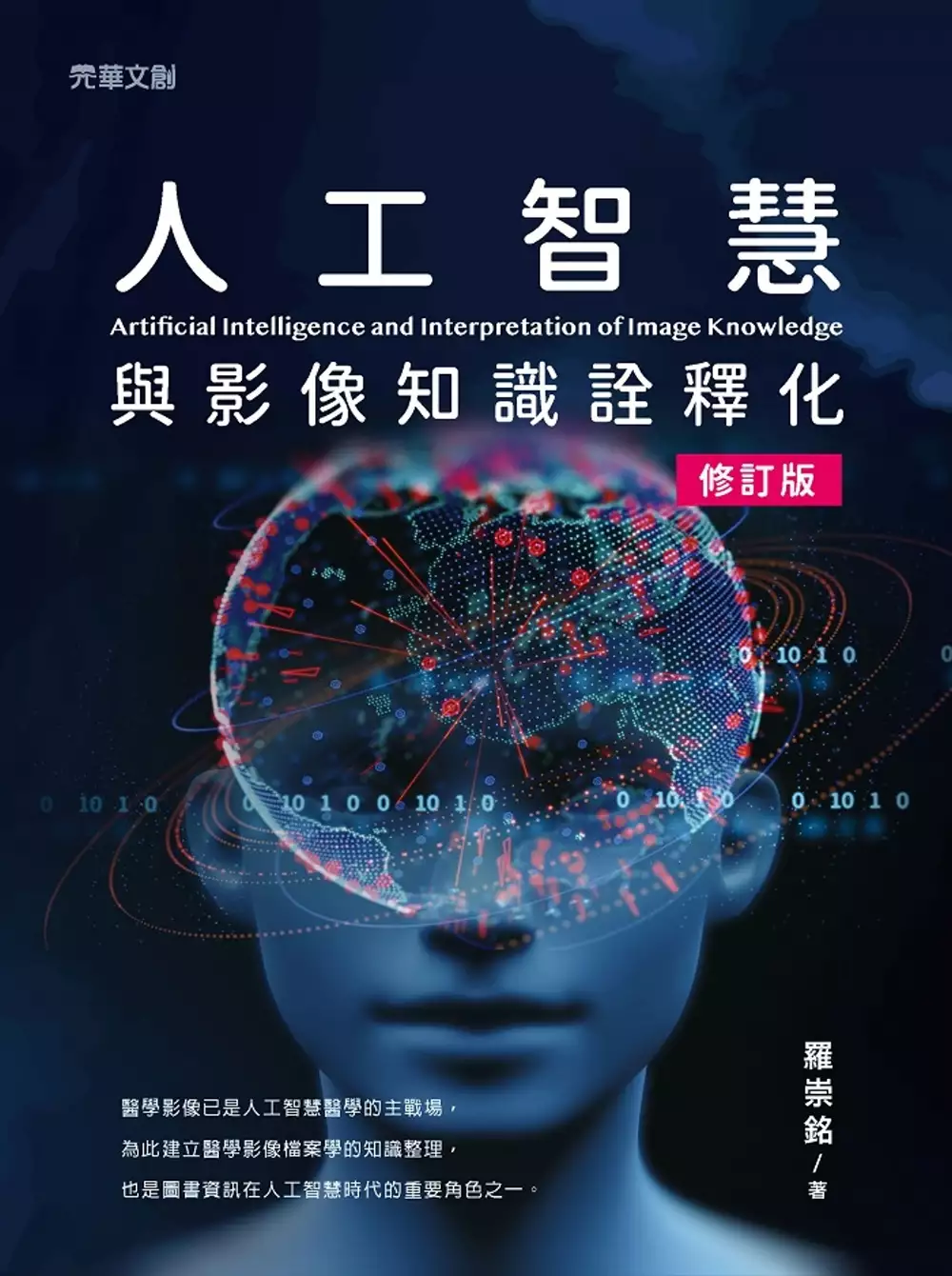
為了解決Bi rads 4 的問題,作者羅崇銘 這樣論述:
本書以資訊數位化出發,跨領域整合醫學影像資訊與圖書資訊,尤其分類與詮釋資料的描述,是資料科學時代相當重要的一環,利用人工智慧進行醫學知識的分類、利用影像特徵的擷取完成影像詮釋資料的建立,並將日益重要的醫學影像的類型加以整理,包括檔案的形成、儲存容量、存取機制、安全性、使用規範、完整性與標註需求,進行鉅細靡遺地闡述,此概念之延伸將有助詮釋與人類生活息息相關的各種影像資料,以完整詮釋建立永恆的知識。 本書特色 醫學影像已是人工智慧醫學的主戰場, 為此建立醫學影像檔案學的知識整理, 也是圖書資訊在人工智慧時代的重要角色之一。
前列腺癌核磁共振影像的直方圖分析與深度卷積神經網絡
為了解決Bi rads 4 的問題,作者賴志慶 這樣論述:
隨著多參數核磁共振影像(mp-MRI)的發展,對於診斷前列腺癌的準確性有所提高,完整的mp-MRI影像包括T2加權影像(T2W)、擴散加權影像(DWI)、擴散係數影像(ADC)和動態對比增強影像(DCE-MRI)。在本論文中,首先利用直方圖參數來分析DCE-MRI影像中對比劑最大洗入斜率(MWS)和延遲洗出斜率(DPS)於攝護腺腫瘤組織與正常組織的區分能力。結果顯示,在攝護腺的過度區,變異係數唯一在MWS中可以區分腫瘤與正常組織的參數,而在DPS中有較多的參數可以區分腫瘤(如 平均值、偏度、10%、25%、50%、75%和90%)。攝護腺外圍區的部分,在MWS分析中,除了峰度和偏度外,其他直
方圖參數對於區分組織,都有顯著的差異。在DPS中有顯著差異的參數為標準差、四分位距、修正過半峰全寬,百分位數距(90%-10%)。在攝護腺的過度區與外圍區的MWS 和 DPS中,有不同的直方圖參數可以被用來區分腫瘤。最後本論文使用以SegNet為基礎的深度卷積神經網路模型來自動分割攝護腺過度區、外圍區和腫瘤的區域。本文中使用PROSTATEx的數據集中的影像來訓練模型,將三種不同序列的核磁共振影像,分別為T2W、DWI和ADC等三種影像,並將其結合為一張三通道(RGB)的影像,並在影像上圈選出三個區域的範圍,最後利用上述三種影像做不同排列的分組來放入模型訓練。結果發現,在T2W + DWI +
ADC影像組合表現出較好的分割效能,而在個別區域分割的相似係數分別為過度區(90.45%)、外圍區(70.04%)和腫瘤區(52.73%),使用深度卷積神經網路模型來診斷腫瘤以及分割區域是相當有潛力的。本文的結論為,對於DCE-MRI 時間強度曲線進行直方圖分析,可以用來區分 過度區與外圍區中的良性前列腺組織和腫瘤,並且深度卷積神經模型於影像序列分析中,用來輔助診斷腫瘤有相當大的潛力。
人工智慧與影像知識詮釋化
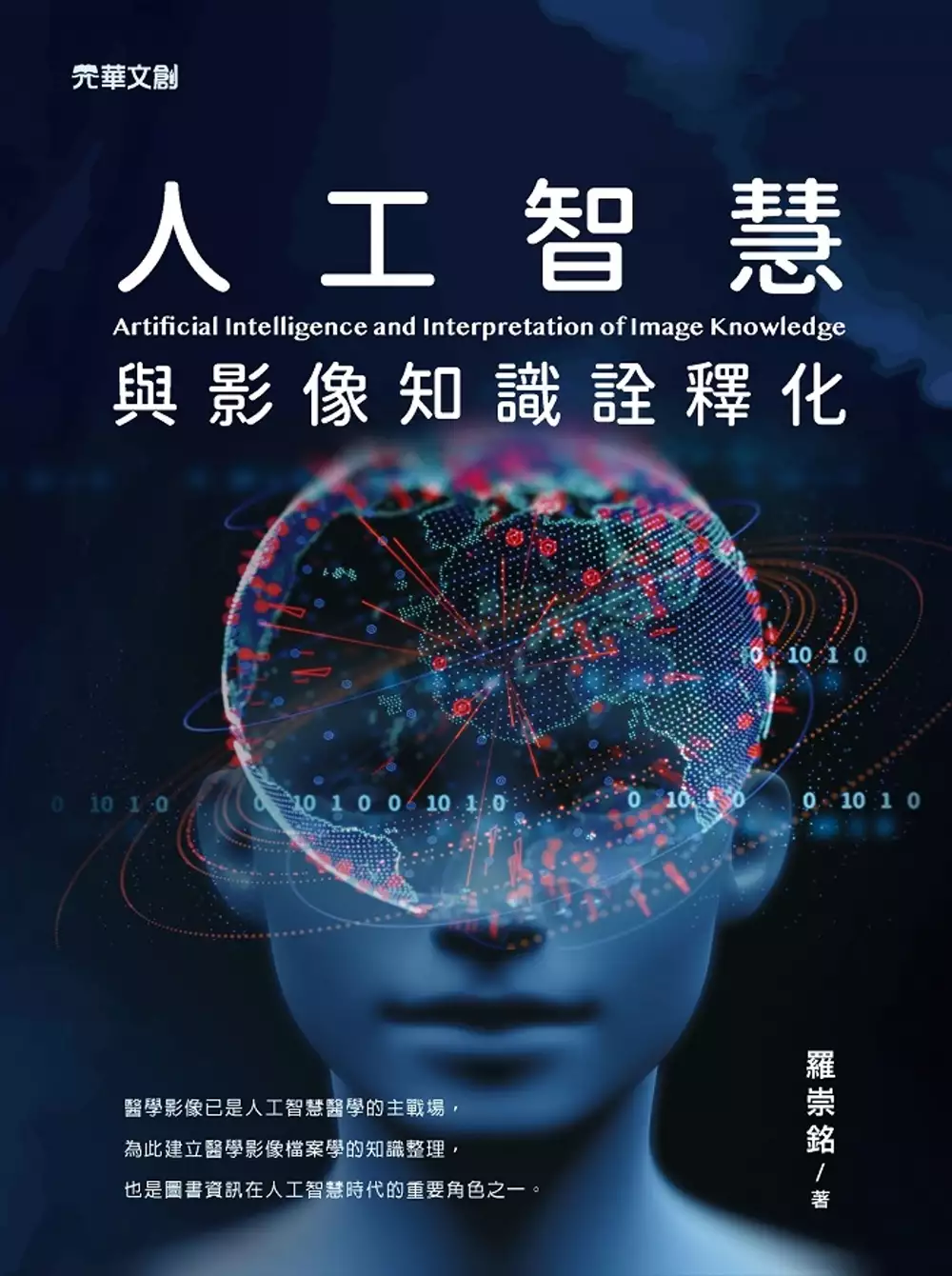
為了解決Bi rads 4 的問題,作者羅崇銘 這樣論述:
本書以資訊數位化出發,跨領域整合醫學影像資訊與圖書資訊,尤其分類與詮釋資料的描述,是資料科學時代相當重要的一環,利用人工智慧進行醫學知識的分類、利用影像特徵的擷取完成影像詮釋資料的建立,並將日益重要的醫學影像的類型加以整理,包括檔案的形成、儲存容量、存取機制、安全性、使用規範、完整性與標註需求,進行鉅細靡遺地闡述,此概念之延伸將有助詮釋與人類生活息息相關的各種影像資料,以完整詮釋建立永恆的知識。 本書特色 醫學影像已是人工智慧醫學的主戰場, 為此建立醫學影像檔案學的知識整理, 也是圖書資訊在人工智慧時代的重要角色之一。
Machine learning algorithm for classification of Ductal carcinoma in situ and minimal invasive breast cancer
為了解決Bi rads 4 的問題,作者VU PHAM THAO VY 這樣論述:
Introduction: Breast cancer nowadays is the second common cancer in the world and the most common cancer among women, excluding nonmelanoma skin cancers. Breast cancer is not just one disease, it has different types and subtypes that depend on the affected specific cell in the breast. Cancer can be
classified into two types according to whether it has spread: non-invasive and invasive breast cancer. The most frequent kind of non-invasive breast cancer is ductal carcinoma in situ (DCIS). DCIS is cancer that starts in a duct and has not spread into any surrounding breast tissue. Some DCIS patie
nts will not develop the invasive disease, and this has been suggested as a risk of screening mammography. Breast cancers that are invasive have grown outside of the ducts or lobules into the surrounding tissue. As size of the tumor decreases, patients with invasive breast cancer have a better chanc
e of surviving. Despite the prognostic factors, a small percentage of patients with invasive tumors of 10 mm or less (T1a and T1b) die from their cancer. Many studies have been conducted examining traditional histopathological characteristics, including lymph node status, tumor size, histological gr
ade, margin width, and many other biological markers of prognosis. The use of these prognostic factors, while appealing in principle and effective in larger tumors, presents challenges in small tumors. The identification of breast cancer types at an early stage enables patients to choose less invasi
ve treatment options. The purpose of our study was to develop a machine-learning classification model to differentiate DCIS and minimal invasive cancer using clinical characteristics, mammography findings, ultrasound findings and histopathology features.Method: Clinical data, mammography findings an
d ultrasound findings of 420 biopsy-confirmed breast cancer cases were analyzed retrospectively to diagnose DCIS and minimal invasive cancer. The subtypes were categorized based on the histopathology and size of lesion on histological assessment. Four groups of features including clinical data, mamm
ography findings, ultrasound findings and histology findings are used for classification by machine learning. The machine learning techniques used in this study include XGboost, Random Forest, Single Vector Machine, Gaussian Naive Bayes, K-Nearest Neighbor, and Decision Tree Classifier. To classify
two types of breast cancer, we mainly focus on the XGBoost algorithm trained on clinical characteristics, mammography (MMG) findings, ultrasound (US) findings, and histopathology features that are associated with DCIS and minimal invasive breast cancers. The study used the area under the receiver op
erating characteristic curve (AUC), sensitivity, specificity, accuracy, precision, and F1 score as measures of model performance. Additionally, this research determined the importance of features by using XGboost and SHapley Additive Explanations (SHAP).Results: The results of this model were valida
ted in 378 women and tested in 42 women (mean age, 58.8 years ± 12.2). The model has high classified performance when combining features importance, with the highest accuracy reaching 0.84 (95% confidence interval [CI]: 0.77, 0.90), an AUC of 0.93 (95% CI: 0.86, 0.96), with the specificity of 0.73 (
95% CI: 0.64, 0.82) and sensitivity of 0.91 (95% CI: 0.73, 0.95). The five most important features illustrated by XGBoost were the presence of calcification on MMG, the existence of lymph node, the presence of microcalcification on histopathology, the shape of the mass on US image, and the orientati
on of mass on US image, and the orientation of mass on US image.Conclusion: XGBoost model combining clinical characteristics, mammography findings, ultrasound findings, and histopathology features, can be applied to classify breast cancer at a level equivalent to radiologists and has the potential t
o detect early invasive breast cancer.
想知道Bi rads 4更多一定要看下面主題
Bi rads 4的網路口碑排行榜
-
#1.Predictive performance of BI-RADS magnetic resonance ...
To determine the positive predictive value (PPV) and likelihood ratio for magnetic resonance imaging (MRI) characteristics of category 4 lesions, as described ... 於 www.scielo.br -
#2.BI-RADS 4是“宣判”乳腺癌了吗
4. BI-RADS 3:良性可能性大的病灶。恶性可能性0%~2%,建议3~6个月后行超声随访复查。 △ 患者,女,39岁 ... 於 m.thepaper.cn -
#3.乳房攝影的解讀系統,BI-RADS
乳房攝影的解讀系統,BI-RADS · 1:正常。 · 2:良性發現。 · 3:可能為良性發現。 · 4:懷疑異常。 · 5:高度懷疑為惡性腫瘤。 · 6:切片已證實為惡性腫瘤。 於 www.femalecancer.org.tw -
#4.Evaluation of the positive predictive value (PPV3) of ACR ...
BI-RADS category 5 is reserved for cancer-like lesions on diagnostic imaging. BI-RADS 4 includes suspicious lesions with some likelihood of malignancy [3,4]. 於 www.polradiol.com -
#5.Compare Deep Learning Features of the Lesion Itself and ...
To determine the feasibility of using a deep learning (DL) approach to identify benign and malignant BI-RADS 4 lesions with preoperative breast DCE-MRI ... 於 www.hindawi.com -
#6.Quais são as alterações classificadas como BI-RADS™ 4?
A categoria BI-RADS 4 significa que existe uma alteração e que se não houver nenhuma contraindicação, uma biópsia deve ser realizada. 於 www.cancerdemamabrasil.com.br -
#7.醫師您好,請問我乳房攝影檢查有照到鈣化,等級是4A
依您描述的內容: 乳房攝影分級4 (BI-RADS 4):懷疑可能惡性發現,應考慮做切片檢查(Suspicious abnormality-Biopsy Should Be Considered),約有3%-94%的機率會成為 ... 於 tw.wen8health.com -
#8.The added value of digital breast tomosynthesis in improving ...
We prospectively evaluated 296 women with BI-RADS indeterminate breast lesions (BI-RADS 0, 3, and 4) by DM between January 2018 and October 2019 ... 於 insightsimaging.springeropen.com -
#9.A Study of BI-RADS 4 and 5 Lesions that are Malignant ...
The purpose of this study is to gather measurements of mechanical properties of BI-RADS 4 and 5 lesions in order to test the effectiveness of MR elastography ( ... 於 www.mayo.edu -
#10.Breast Imaging - Google 圖書結果
If follow-up imaging surveillance reveals the focal asymmetry has developed suspicious features, then an imaged-guided biopsy is warranted (BI-RADS 4). 於 books.google.com.tw -
#11.Positive Predictive Value Assessment of Malignancy in BI- ...
In this classification, BI-RADS category 4 is reserved for imaging findings that do not have the classic features of malignancy but are ... 於 www.iomcworld.org -
#12.怎麼看懂乳房攝影報告?先看分級、再看乳腺緻密程度「這情況 ...
BI -RADS 0:乳房有變化,但是單以乳房攝影無法判斷,需再進行其他檢查。 BI-RADS 1:乳房正常。 ... BI-RADS 4:乳房有異常變化,可能是惡性的。 於 health.tvbs.com.tw -
#13.Results of Short-Term Follow-Up in BI-RADS 3 and 4a ...
The lesions are then classified as BI-RADS 4, and histological characterization is mandatory. When a biopsy is performed, the risk of incomplete ... 於 karger.com -
#14.Surgery: A Case Based Clinical Review - 第 49 頁 - Google 圖書結果
Workup 5 BI-RADS (0–5) is a standardized summary of mammographic findings. 5 BI-RADS 3 is probably benign (2% chance of cancer); BI-RADS 4 is suspicious ... 於 books.google.com.tw -
#15.Diffusion-weighted Imaging Allows for Downgrading MR BI ...
BI -RADS 4 Lesions in Contrast-enhanced MRI of the. Breast to Avoid Unnecessary Biopsy. Paola Clauser1, Barbara Krug2, Hubert Bickel1, ... 於 aacrjournals.org -
#16.乳腺超声报告BI-RADS:4级,59岁高新区居民转诊 ...
BI -RADS 4级:可能恶性的病变,可细分为4A、4B、4C。 一般而言,那些看起来像腺瘤,比如有1项次要恶性特征,如边缘模糊等,则评估为4a, ... 於 www.zxyy.cn -
#17.乳篩結果BI-RADS 0或4 快回醫院複檢 - 元氣網
乳癌堪稱女性健康殺手,每年都有超過萬名新發乳癌,發生率高居亞洲之冠,死亡率則為女性癌症第4位,政府雖提供女性免費乳癌篩檢... 於 health.udn.com -
#18.BI-RADS 4 and 5 breast lesions
BI -RADS 4 and 5 breast lesions: correlation between sonographic findings and histopathological results following ultrasound-guided FNAC. يدﺛﻟا تﺎﻓآ. 於 www.iasj.net -
#19.Dr. Breast專欄: 【乳房攝影報告Q&A】
乳房攝影結果BIRADS分類:美國放射線學會將乳房攝影結果訂為六個分級, ... 當被通知為BI-RADS 3、4、5,請一定要依照醫師囑咐追蹤或複檢,乳癌早期 ... 於 www.nbic.com.tw -
#20.乳房檢查報告的判讀- 簡介BIRADS
4. BIRADS 3:可能為良性發現。如未鈣. 化且界限清楚的實心腫塊、局部不對稱 ... BIRADS 4可再區分為4A、4B. 和4C。 (1) 4A主要是指可觸摸得到且界限清楚. 於 www.tafm.org.tw -
#21.亞東院訊
BI -RADS分類. 臨床意義. 0. 結果未能確定,需進一步檢查。 1. 無異常發現。 2. 良性病灶。 3. 可能是良性,需再追蹤檢查。(惡性機率≤2%). 4. 可疑。 於 www.femh.org.tw -
#22.经乳腺专用MRI诊断为BI-RADS 4类病灶良恶性比例及“误诊” ...
方法:收集我院经DBMRI诊断为BI-RADS 4类的781例患者的资料,参照美国放射学BI-RADS分类标准,结合我院临床经验,认为形态学及血流动力学两项指标,一项表现为非良性,则将病灶归 ... 於 www.qk.sjtu.edu.cn -
#23.SEER Inquiry System - Question 20210075 Details
–Prostate cases with a PI-RADS category 4 or 5 (reportable since 2017) ... –Breast cases designated BI-RADS 4, 4A, 4B, 4C or BI-RADS 5. 於 seer.cancer.gov -
#24.Bi-RADS for Mammography and Ultrasound 2013
The ACR BI-RADS Atlas 2013 (4) is the updated version of the 2003 Atlas. BI-RADS® is designed to standardize breast imaging reporting and to ... 於 radiologyassistant.nl -
#25.TNM Staging and BI-RADS Grading for Breast Cancer
The letters T followed by a number between 0 and 4 indicate the extent to which the primary tumour has spread to the skin and the chest wall. Larger tumours or ... 於 www.pacehospital.com -
#26.BI-RADS分級- 乳房健康
1至3等級是安全的狀態,而0,4,5三種類別可能會被要求進行覆檢。 各項乳房健康詳情如下: 乳腺疾病 · 乳腺增生 · 乳腺炎 · 乳腺囊腫 · 乳癌 ... 於 www.combinedwomen.hk -
#27.Positive Predictive Value of Malignancy in BI-RADS 4 and ...
Objective: To determine the positive predictive value (PPV) of the breast imaging and data system. (BI-RADS) category 4 and 5 in diagnosis of breast cancer. The ... 於 he02.tci-thaijo.org -
#28.Birads 4 en una mamografía ¿Es Cáncer?
La categoría BI-RADS 4 en el informe de una mamografía significa que se ha observado un hallazgo sospechoso. Aunque no es una imagen con ... 於 www.diagnosticorojas.com.ar -
#29.Impact of the Kaiser score on clinical decision-making in BI ...
As the database cases were diagnosed during assessment, breast cancer screening, and curative mammography, no subdivision into BI-RADS 4 a–c ... 於 link.springer.com -
#30.磁共振成像对乳腺BI-RADS 4类病变诊断效能的研究
目的. 探讨MRI对美国放射学院(American College of Radiology, ACR)乳腺影像报告与数据系统(Breast Imaging Reporting and Data System, BI-RADS)4类 ... 於 rs.yiigle.com -
#31.看不懂乳房攝影報告怎麼辦?醫師教從七個分級判斷是否回診
BI -RADS 3:乳房有變化,可能是良性的。建議短期追蹤。 BI-RADS 4:乳房有異常變化,可能是惡性的。需考慮進一步切片確認。 於 tw.tech.yahoo.com -
#32.如何早期發現乳癌? - 台灣癌症防治網
BIRADS 的分級包含了0,1,2,3,4,5,6共七個等級,他們所代表的意義簡單說明如下:. 1.正常(normal). 2.良性(benign). 3.可能良性(probably benign). 4.懷疑(suspicious). 於 web.tccf.org.tw -
#33.Encyclopedia of Imaging - 第 133 頁 - Google 圖書結果
Applications and literature review of the BI-RADS classification. ... US-BI-RADS (4) Features in ultrasound according to the BI-RADS classification are ... 於 books.google.com.tw -
#34.Assessment of symptomatic BI-RADS 4 in tertiary care ...
6. According to one study, most BI-RADS 4 lesions revealed fibrocystic modification on histological evaluation followed by ductal carcinoma in ... 於 medcraveonline.com -
#35.Utility of BI-RADS Assessment Category 4 Subdivisions for ...
OBJECTIVE. BI-RADS for mammography and ultrasound subdivides category 4 assessments by likelihood of malignancy into categories 4A (> 2% to ... 於 www.ajronline.org -
#36.Assessment of BI-RADS Category 4 Lesions Detected with ...
It is well known, however, that imaging findings classified as BI-RADS category 4 cover a wide range of malignancy rates; the current BI-RADS ... 於 pubs.rsna.org -
#37.多模态超声成像评分法在BI-RADS 4类乳腺肿块中的应用价值
方法:选取189个灰阶超声(US)诊断为BI-RADS 4类乳腺肿块,病理结果均由手术方式获得。所有肿块均经微血管血流成像、弹性超声获得血管指数(VI)、弹性应变比(SR)定量参数 ... 於 hanspub.org -
#38.Evaluation of Real-world Data for Breast-MRI BI-RADS IV ...
In 2013, the American College of Radiology released a newer BI-RADS 4 classification. BI-RADS 4 is now divided into category 4a (risk of malignancy 2-10%), ... 於 iv.iiarjournals.org -
#39.Breast cancer: Global quality care - 第 116 頁 - Google 圖書結果
BI -RADS 4: Suspicious Management: tissue diagnosis. Comment: intermediate risk of malignancy. Appropriate action to be taken (usually percutaneous biopsy). 於 books.google.com.tw -
#40.BI-RADS分级是什么意思?教你看懂「乳腺钼靶」检查报告单
4. 其它征象. 如局部皮肤增厚,既可能是良性疾病,也可能是恶性疾病。 恶性疾病包括炎性乳腺癌 ... 於 zhuanlan.zhihu.com -
#41.37. 一位45 歲女性接受篩檢乳房攝影( screening mam..
BIRADS 4 suspicious abnormality, 需依病灶的情況進一步做細或粗針穿刺或切片檢查, ... 顯示低回音病灶(hypoechoic lesion),檢查結果報告為BI-RADS Category: 4。 於 twmlestep2.appspot.com -
#42.AI proves comparable to rads for assessing BI-RADS 4 ...
An AI model using breast ultrasound images is on par with human experts when it comes to assessing BI-RADS 4 lesions. 於 www.auntminnie.com -
#43.乳房鈣化點- 係咪好危險?-香港的乳癌治療資訊 - Breast HK
請問照出係birads 4 ,係惡性機會好大,謝謝。 Reply. Breast HK September 26. 2-95%. 於 www.breasthk.com -
#44.看不懂乳房攝影報告怎麼辦?醫師教從七個分級判斷是否回診
BI -RADS 0、4 、 5:通常還需要其他的檢查才能確認診斷,請盡快回乳房外科門診就診,王亭雅提醒:「回診時請記得帶書面報告跟影像的光碟片,才能讓醫師有更好的判斷。 於 heho.com.tw -
#45.Breast imaging-reporting and data system (BI-RADS) ...
BI -RADS 4 lesions may not have the characteristic morphology of breast cancer but have a definite probability of being malignant. A biopsy is ... 於 radiopaedia.org -
#46.Assessing the Positive Predictive Value of Architectural ...
Tomosynthesis-detected BI-RADS 4 AD are malignant in 35% of cases and are more likely to be malignant if an US correlate is present and if ... 於 academic.oup.com -
#47.BI-RADS 4 breast lesions: could multi-mode ... - Gland Surgery
Background: The malignant probability of Breast Imaging Reporting and Data System (BI-RADS) 4 breast lesions is 3–94%, which is a very large span, ... 於 gs.amegroups.org -
#48.Understanding Your Mammogram Report
What do the BI-RADS categories mean? ; 4. Suspicious abnormality – Biopsy should be considered. These findings do not definitely look like cancer ... 於 www.cancer.org -
#49.BI-RADS
A BI-RADS classification of 4 or 5 warrants biopsy to further evaluate the offending lesion. Some experts believe that the single BI-RADS 4 classification does ... 於 en.wikipedia.org -
#50.乳房X光影像BI-RADS分類與嚴重程度排序
人工智慧在醫學影像分析(四):乳房X光影像BI-RADS分類與嚴重程度排序 ... BI-RADS分級具有困難性,醫師必須檢視單一個案的多張乳房影像,從影像中分析 ... 於 www.find.org.tw -
#51.Nonpalpable BI-RADS 4 breast lesions
Abstract. Purpose: We aimed to evaluate ultrasonography (US) findings for Breast Imaging Reporting and Data System (BI-RADS) category 4 lesions using ... 於 pubmed.ncbi.nlm.nih.gov -
#52.BI-RADS Classification for Management of Abnormal ...
(J Am Board Fam Med 2006;19:161–4.) The Breast Imaging Reporting and Data System. (BI-RADS) was developed in 1993 by the American. College of Radiology ... 於 www.jabfm.org -
#53.X 线钼靶与超声引导下穿刺活检在BI-RADS4类乳腺肿块鉴别 ...
结果:在84个BI-RADS 4类乳腺肿块病灶中,恶性48个,良性36个。超声弹性成像的诊断敏感度、特异度、准确度、阳性预测值、阴性预测值、漏诊率、误诊率分别为95.8 ... 於 www.zpwz.net -
#54.Understanding BI-RADS | Barnes-Jewish St. Peters Hospital
BI -RADS 4: This means the findings on your study are suspicious, and there is an approximately 23 to 34 percent chance of breast cancer. 於 www.bjsph.org -
#55.What to Know About Your BI-RADS Score
Category 4: There is a suspicious abnormality that may be cancerous. This result requires a biopsy. Category 5: The growth is highly likely to ... 於 www.webmd.com -
#56.Understanding BI-RADS for Breast MR
We analyzed data from 8,283 breast MR exams from four regional registries in the Breast Cancer Surveillance Consortium (BCSC) for the years 2005 ... 於 radiology.ucsf.edu -
#57.correlation between ultrasound bi-rads 4 breast lesions ...
Keywords: BIRADS 4 breast lesion, ultrasound, fine needle aspiration cytology. SAŽETAK. Sažetak: Kancer dojke je najuobičajenija vrsta maligniteta kod žena i ... 於 www.sciendo.com -
#58.Predictive Value of BI-RADS Category 4A and 4B Lesions ...
Among these categories, category 4 is suspected to have malignant changes, and biopsy is recommended. The risk of cancer is between 2 and 94% ... 於 www.jsu.or.kr -
#59.乳癌篩檢初體驗(上)
報告上顯示的是 BI-RADS 4 ... BI-RADS 4 是有可疑惡性.. 建議活檢(做切片檢查) ... 4/16 下午,到醫院做超音波導引定位+穿刺檢查(組織切片). 於 misswindchen2013.pixnet.net -
#60.BI-RADS 4类乳腺癌的超声图像特征分析
BI -RADS 4类乳腺癌的超声图像特征分析. 梁新凤,吴林生,沈晓临,吴建梅,王艳丽. 上海市黄浦区中心医院超声科,上海200002. Ultrasound images of BI-RADS category 4 ... 於 www.zhongliuyingxiangxue.com -
#61.禾馨民權婦幼診所- 如何了解乳房影像判讀書面報告
美國放射科醫學會(ACR)公佈一套乳房影像報告系统(BI-RADS),將乳房X光攝影、乳房超音波、乳房磁振造影(MRI)的檢查結果標準化,建立起醫師間及醫師與受檢者間的 ... 於 www.dianthus.com.tw -
#62.Surprise, You Have Early Breast Cancer
The results came back that I had the most dense tissue and that I fell into a category called BI-RADS 4, meaning that something could be ... 於 www.nytimes.com -
#63.Atlas of breast cancer early detection
BI -RADS 4 indicates suspicious mammography findings that need further biopsy for confirmation. BI-RADS 4 lesions do not show morphological features ... 於 screening.iarc.fr -
#64.衛生福利部【台灣e院】-乳房甲狀腺科 常見問題
6公分大的腫瘤無法像之前4公分時一樣用局部麻醉處理嗎? ... BI-RADS 4A 馬上就做了超音波四次粗針的切片下個禮拜回去看報告醫生說是良性的順便加做乳房攝影乳房攝影的 ... 於 sp1.hso.mohw.gov.tw -
#65.乳房篩檢與自我檢查- 嘉義長庚一般外科
乳房攝影篩檢疑陽個案(如:BI-RADS category 0,4,5)須進一步確診者,除進一步的診斷性乳房攝影術外,超音波也扮演重要的角色。 疑似發炎或感染性乳房病兆,超音波掃描 ... 於 www1.cgmh.org.tw -
#66.Reducing the number of unnecessary biopsies of US-BI- ...
Breast lesions classified into BI-RADS 4a were defined as having low suspicion for malignancy. In the clinical setting, category 4a is a relatively complicated ... 於 bmjopen.bmj.com -
#67.Reducing the number of unnecessary biopsies for ...
In clinical practice, the diagnosis of BI-RADS category 4 can be influenced by the clinical experience of the radiologist, particularly that of ... 於 bmcmedimaging.biomedcentral.com -
#68.A Comparative Assessment of MR BI-RADS 4 Breast ...
The probability of malignancy of BI-RADS 4 lesions varies from 2% to 95% (5, 7), indicating that a large number of benign lesions would receive ... 於 www.frontiersin.org -
#69.A Nomogram Combining Radiomics and BI-RADS
Category 4 is defined as suspicious lesion with 2% to 95% malignant probability that is recommended for biopsy. Category 5 is defined as highly ... 於 www.nature.com -
#70.Comparative accuracy of sonography, mammography and ...
Breast masses under BI-RADS 4 are not classically malignant, but are suspicious enough for core needle biopsy while BI-RADS 5 masses have higher ... 於 www.joghr.org -
#71.bi rads 4的原因和症狀, 台灣e院的回答
BI -RADS category 4 means there is a suspicious abnormality on your breast imaging studies and a biopsy should be considered as a next step. 於www.dic-kc.com. # ... 於 hospice.mediatagtw.com -
#72.BI-RADS 4 subcategories | Download Table
Breast Imaging Reporting and Data Systems (BI- RADS) 4 assessment is given to lesions that carry a risk of malignancy between 2 and 95 % and, in the United ... 於 www.researchgate.net -
#73.RESEARCH ARTICLE Prediction for Breast Cancer in BI ...
as BI-RADS category 4 and subcategories 4a, 4b, 4c. A total of 961 breast lesions consisted of 772 (80.33%) benign. 於 journal.waocp.org -
#74.臺大醫療體系乳癌篩檢陽性不複檢之原因探討
在臺灣,乳癌篩檢已經行之有年,攝影篩檢結果為BI-RADS 0和BI-RADS 4的民眾,會被以郵件通知前來複檢。 然而,這些民眾的回診率只有八成左右,大約兩成具乳癌高風險的 ... 於 epaper.ntuh.gov.tw -
#75.Guide to Mammography Reports: BI-RADS Terminology
BI -RADS category 4 includes any other findings for which malignancy cannot be excluded, ranging pathologically from fibrocystic tissue to cancer ... 於 www.aafp.org -
#76.運用機器學習改善乳房超音波彈性影像判讀準確性
依據美國放射學會發布的乳房影像報告與資料系統,可以知道BI-RADS 3、4 級之間為腫瘤良惡性的分界線。本研究從超音波彈性影像運用一系列影像處理技術偵測出腫瘤與其 ... 於 ndltd.ncl.edu.tw -
#77.A comparative efficacy study of diagnostic digital breast ...
We compare clinical performance of digital breast tomosynthesis (DBT) versus digital mammography (2D) among our BI-RADS 4 population without ... 於 www.ejradiology.com -
#78.Breast Follow-up Algorithms
CBE every 1-3 years. Age 40 or older: Return to annual CBE and screening mammography. *Consider tomosynthesis. NOTE. Mammogram Findings: BI-RADS 4 OR 5. 於 www.michigan.gov -
#79.MRI for the assessment of malignancy in BI-RADS 4 ...
Reported disease prevalence for BI-RADS 4 mammographic microcalcifications range from 32 to 65.2% and from 91.4 to 100% for BI-RADS 5 rated lesions [5]. 於 journals.plos.org -
#80.Mammogram Results and BI-RADS Score Category
Category 4 means the findings suspect cancer. Category 4 has three subcategories based on the chance of cancer: ... Follow-up care calls for careful monitoring ... 於 www.cancercenter.com -
#81.A Deep Learning–Based Decision Support Tool for ...
Therefore, we developed the Breast Cancer Risk Calculator (BRISK) to target a well-characterized and specific patient subgroup (BI-RADS 4) ... 於 ascopubs.org -
#82.DBT not the superior modality for assessing BI-RADS 4 ...
“Among the BI-RADS categories, BI-RADS 4—“suspicious findings with a recommendation for biopsy”—stands out for its enormous uncertainty, with a ... 於 healthimaging.com -
#83.乳房鈣化點- 乳癌- 癌症簡介- 癌症防治- 民眾服務
例如:報告結果為BIRADS 4,則罹患乳癌的可能性增加為125倍。 乳房鈣化點評估. 類別. 評估結果. 乳癌可能性(百分比). 乳癌 ... 於 www.cgh.org.tw -
#84.Journal of the National Cancer Institute: JNCI.
Women with BI - RADS 4 readings were the youngest and least likely to use HRT compared with women in all other groups . Restricting comparisons to women ... 於 books.google.com.tw -
#85.女性常用乳房腫瘤檢查(上)
BI -RADS 3:可能良性。其惡性腫瘤的風險低於2%. ,建議短期追蹤。 BI-RADS 4:懷疑異常。 於 www.tmuh.org.tw -
#86.Breast Imaging Reporting & Data System (BI-RADS®)
The BI-RADS atlas includes: Over 700 clinical images; Follow-up and outcome monitoring includes mammography, ultrasound and MRI; Updated breast composition ... 於 www.acr.org -
#87.Mammogram Reports and BI-RADS: Categories 4 & 5
BI -RADS category 4 means there is a suspicious abnormality on your breast imaging studies and a biopsy should be considered as a next step. 於 www.dic-kc.com -
#88.Birads 4 and 5 Categories: The chances of cancer
Generally speaking, if a radiologist feels that a mammogram corresponds to BI-RADS category 4, it probably means, in their own mind, that they ... 於 breast-cancer.ca -
#89.BI-RADS Score: Results Explained - YouTube
When I had my first mammogram, the radiologist put me at BIRADS 4 for a suspicious looking intraductal papilloma. 於 www.youtube.com -
#90.A New Practical Decision Rule to Better Differentiate BI ...
A patient should undergo a diagnostic biopsy (BI-RADS 4) instead of follow-up imaging (BI-RADS 3) if the patient is 50 years or older or ... 於 onlinelibrary.wiley.com -
#91.A Practical Guide to Understanding BIRADS
[DocPanel] A BIRADS 4 indicates a suspicious finding with a 2-94% probability of malignancy. That's a broad range. What advice would you give a ... 於 www.docpanel.com -
#92.Accuracy of subcategories A, B, C in BI-RADS 4 lesions by ...
Accuracy of subcategories A, B, C in BI-RADS 4 lesions by combined mammography and breast ultrasound findings · Figures and Tables · 9 Citations · 19 References ... 於 www.semanticscholar.org -
#93.A Five-Year Review of the Outcomes of Breast Imaging ...
Among those, BI-RADS 4 is associated with a wider range of risk for breast cancer, which makes the decision for biopsy difficult. The study aim ... 於 www.cureus.com -
#94.Dedicated breast PET value to evaluate BI-RADS 4 ...
According to the American College of Radiology Breast Imaging-Reporting and Data System lexicon [1], category 4 assessment includes suspicious lesions, with a ... 於 www.sciencedirect.com -
#95.Value of the US BI-RADS final assessment following ...
BI -RADS category 4a was defined for lesions with one or more minor suspicious findings, category 4b for lesions showing more than three minor suspicious ... 於 journals.sagepub.com -
#96.Atlas of Breast Tomosynthesis: Imaging Findings and ...
3.58a, b [3D magnification, same figure as 3.58a]); BI-RADS 4. Differential Diagnosis on 2D Cyst, lymph node, fibroadenoma, DCIS, invasive breast cancer ... 於 books.google.com.tw