bi-rads的問題,我們搜遍了碩博士論文和台灣出版的書籍,推薦羅崇銘寫的 人工智慧與影像知識詮釋化(修訂版) 和羅崇銘的 人工智慧與影像知識詮釋化都 可以從中找到所需的評價。
另外網站Practical and illustrated summary of updated BI-RADS for ...也說明:The American College of Radiology (ACR) fifth edition of the Breast Imaging-Reporting and Data. System [BI-RADS] Atlas [1] provides ...
這兩本書分別來自元華文創股份有限公司 和元華文創股份有限公司所出版 。
臺北醫學大學 國際醫學研究碩士學位學程 陳榮邦所指導 VU PHAM THAO VY的 Machine learning algorithm for classification of Ductal carcinoma in situ and minimal invasive breast cancer (2021),提出bi-rads關鍵因素是什麼,來自於Ductal carcinoma in situ (DCIS)、minimal invasive breast cancer、machine learning、ultrasound imaging、mammographic imaging。
而第二篇論文國立臺中科技大學 護理系 怡懋·蘇米所指導 曾家琪的 從社會資本理論探討乳癌篩檢異常個案回診率之相關研究 (2021),提出因為有 乳癌篩檢、乳房攝影、再回診、回診率、社會資本的重點而找出了 bi-rads的解答。
最後網站BI-RADS Category 5 Assessments at Diagnostic Breast ...則補充:Rationale and Objectives The Breast Imaging-Reporting and Data System (BI-RADS) atlas defines category 5 assessments as appropriate only for lesions that ...
人工智慧與影像知識詮釋化(修訂版)
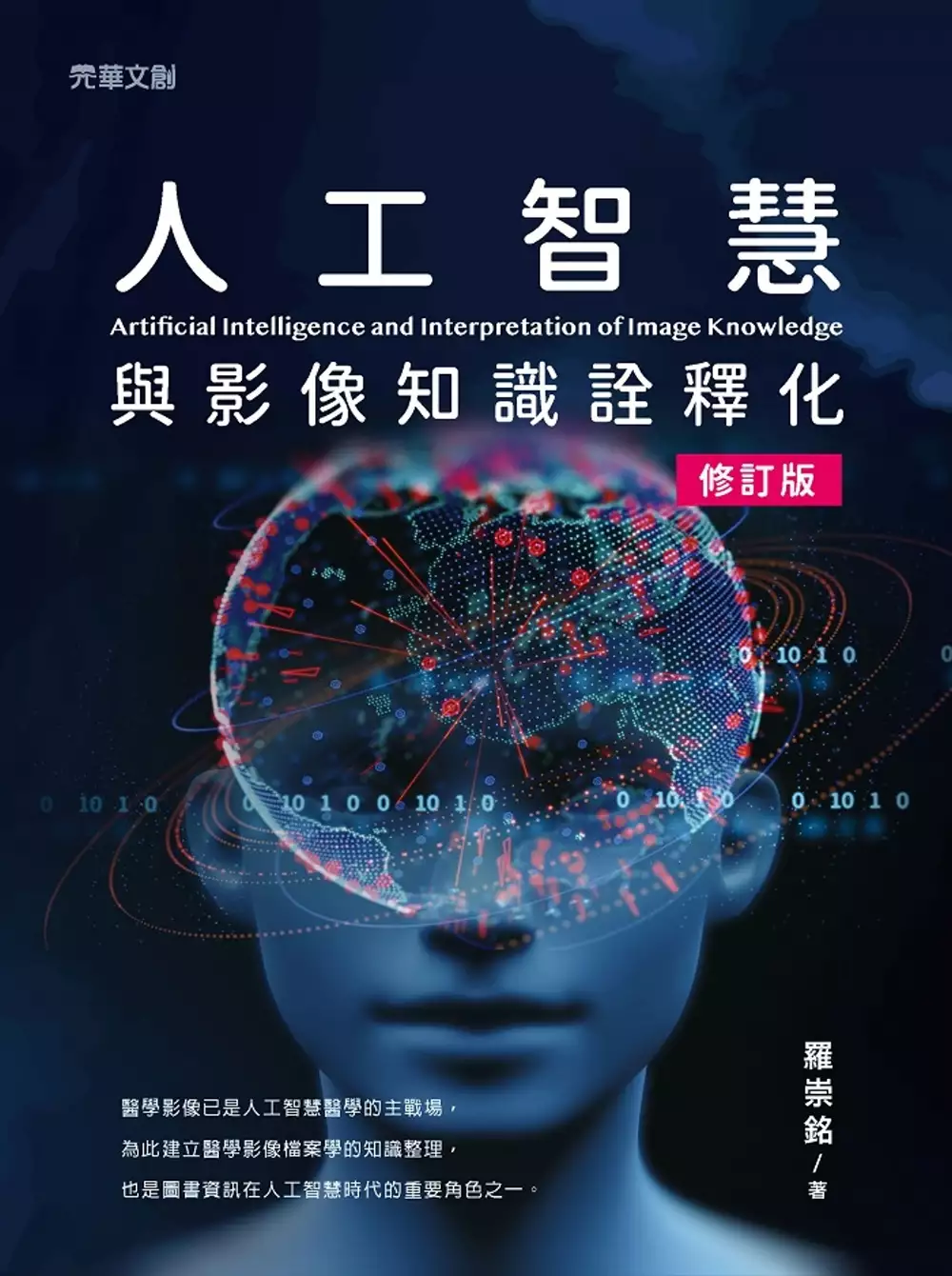
為了解決bi-rads 的問題,作者羅崇銘 這樣論述:
本書以資訊數位化出發,跨領域整合醫學影像資訊與圖書資訊,尤其分類與詮釋資料的描述,是資料科學時代相當重要的一環,利用人工智慧進行醫學知識的分類、利用影像特徵的擷取完成影像詮釋資料的建立,並將日益重要的醫學影像的類型加以整理,包括檔案的形成、儲存容量、存取機制、安全性、使用規範、完整性與標註需求,進行鉅細靡遺地闡述,此概念之延伸將有助詮釋與人類生活息息相關的各種影像資料,以完整詮釋建立永恆的知識。 本書特色 醫學影像已是人工智慧醫學的主戰場, 為此建立醫學影像檔案學的知識整理, 也是圖書資訊在人工智慧時代的重要角色之一。
bi-rads進入發燒排行的影片
Machine learning algorithm for classification of Ductal carcinoma in situ and minimal invasive breast cancer
為了解決bi-rads 的問題,作者VU PHAM THAO VY 這樣論述:
Introduction: Breast cancer nowadays is the second common cancer in the world and the most common cancer among women, excluding nonmelanoma skin cancers. Breast cancer is not just one disease, it has different types and subtypes that depend on the affected specific cell in the breast. Cancer can be
classified into two types according to whether it has spread: non-invasive and invasive breast cancer. The most frequent kind of non-invasive breast cancer is ductal carcinoma in situ (DCIS). DCIS is cancer that starts in a duct and has not spread into any surrounding breast tissue. Some DCIS patie
nts will not develop the invasive disease, and this has been suggested as a risk of screening mammography. Breast cancers that are invasive have grown outside of the ducts or lobules into the surrounding tissue. As size of the tumor decreases, patients with invasive breast cancer have a better chanc
e of surviving. Despite the prognostic factors, a small percentage of patients with invasive tumors of 10 mm or less (T1a and T1b) die from their cancer. Many studies have been conducted examining traditional histopathological characteristics, including lymph node status, tumor size, histological gr
ade, margin width, and many other biological markers of prognosis. The use of these prognostic factors, while appealing in principle and effective in larger tumors, presents challenges in small tumors. The identification of breast cancer types at an early stage enables patients to choose less invasi
ve treatment options. The purpose of our study was to develop a machine-learning classification model to differentiate DCIS and minimal invasive cancer using clinical characteristics, mammography findings, ultrasound findings and histopathology features.Method: Clinical data, mammography findings an
d ultrasound findings of 420 biopsy-confirmed breast cancer cases were analyzed retrospectively to diagnose DCIS and minimal invasive cancer. The subtypes were categorized based on the histopathology and size of lesion on histological assessment. Four groups of features including clinical data, mamm
ography findings, ultrasound findings and histology findings are used for classification by machine learning. The machine learning techniques used in this study include XGboost, Random Forest, Single Vector Machine, Gaussian Naive Bayes, K-Nearest Neighbor, and Decision Tree Classifier. To classify
two types of breast cancer, we mainly focus on the XGBoost algorithm trained on clinical characteristics, mammography (MMG) findings, ultrasound (US) findings, and histopathology features that are associated with DCIS and minimal invasive breast cancers. The study used the area under the receiver op
erating characteristic curve (AUC), sensitivity, specificity, accuracy, precision, and F1 score as measures of model performance. Additionally, this research determined the importance of features by using XGboost and SHapley Additive Explanations (SHAP).Results: The results of this model were valida
ted in 378 women and tested in 42 women (mean age, 58.8 years ± 12.2). The model has high classified performance when combining features importance, with the highest accuracy reaching 0.84 (95% confidence interval [CI]: 0.77, 0.90), an AUC of 0.93 (95% CI: 0.86, 0.96), with the specificity of 0.73 (
95% CI: 0.64, 0.82) and sensitivity of 0.91 (95% CI: 0.73, 0.95). The five most important features illustrated by XGBoost were the presence of calcification on MMG, the existence of lymph node, the presence of microcalcification on histopathology, the shape of the mass on US image, and the orientati
on of mass on US image, and the orientation of mass on US image.Conclusion: XGBoost model combining clinical characteristics, mammography findings, ultrasound findings, and histopathology features, can be applied to classify breast cancer at a level equivalent to radiologists and has the potential t
o detect early invasive breast cancer.
人工智慧與影像知識詮釋化
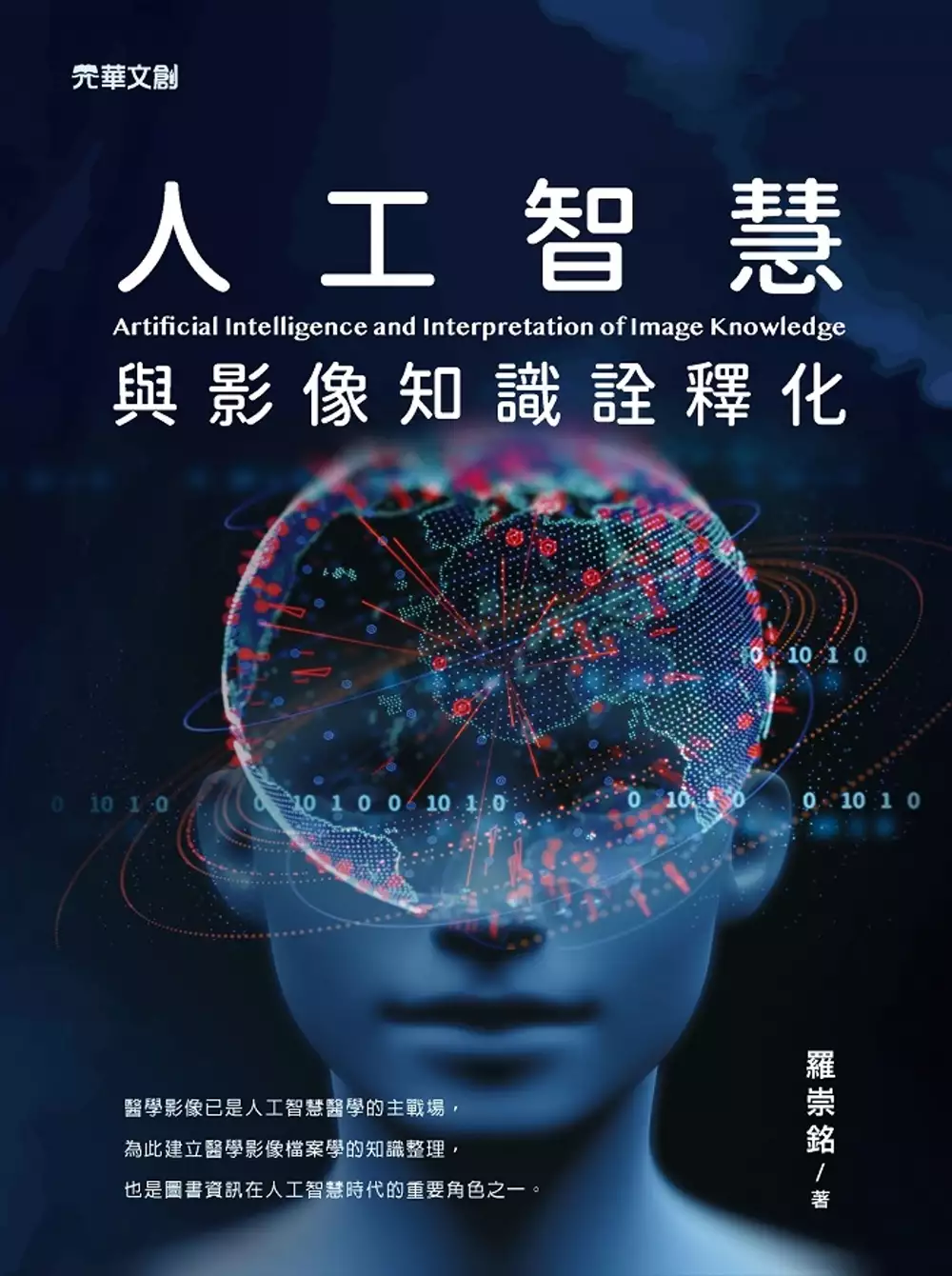
為了解決bi-rads 的問題,作者羅崇銘 這樣論述:
本書以資訊數位化出發,跨領域整合醫學影像資訊與圖書資訊,尤其分類與詮釋資料的描述,是資料科學時代相當重要的一環,利用人工智慧進行醫學知識的分類、利用影像特徵的擷取完成影像詮釋資料的建立,並將日益重要的醫學影像的類型加以整理,包括檔案的形成、儲存容量、存取機制、安全性、使用規範、完整性與標註需求,進行鉅細靡遺地闡述,此概念之延伸將有助詮釋與人類生活息息相關的各種影像資料,以完整詮釋建立永恆的知識。 本書特色 醫學影像已是人工智慧醫學的主戰場, 為此建立醫學影像檔案學的知識整理, 也是圖書資訊在人工智慧時代的重要角色之一。
從社會資本理論探討乳癌篩檢異常個案回診率之相關研究
為了解決bi-rads 的問題,作者曾家琪 這樣論述:
因乳癌發生率為國內女性癌症首位,死亡率為第四名,乳癌篩檢的觀念愈來愈受到重視,影響乳癌篩檢陽性個案再回診的社會資本也相形重要,過去研究證實社會資本會影響健康,甚至與死亡率的風險因素有明確關係。因此,本研究主要探討乳癌篩檢個案從發現到異常、就醫和確診與社會資本之關係,運用社會資本理論探討乳癌篩檢異常個案回診率的影響。本研究為調查性研究,以中部某教學醫院之衛教癌症篩檢中心,以G-power估算收案人數共105人,收案對象為 45-70 歲乳癌篩檢異常之個案為研究對象,以「社會資本」為問卷主題內容,其中包括社會支持、社會參與、社會信任三個面向,每一構面以三項指標來做相關及差異性分析,所得資料以 S
PSS24.0 進行描述性統計及推論性統計。研究結果發現到乳癌篩檢異常個案在自我決策、家人關係、同事及朋友關係醫療人員關係顯示結果較高者,乳癌篩檢異常個案之回診率有明顯提升,反之則是下降的,當中又以社會資本裡的醫療人員關係,為乳癌篩檢異常個案再回診率之重要預測因子;目前國內尚無文獻針對乳癌篩檢異常與社會資本的兩者間提出臨床實務成效及評價求醫歷程,本文旨在研究以社會資本在乳癌篩檢異常個案回診率之相關研究,檢視其適用性和衛教成效,以提出社會資本與乳癌篩檢異常個案提升回診率之臨床實務應用。
想知道bi-rads更多一定要看下面主題
bi-rads的網路口碑排行榜
-
#1.Making the Diagnosis: A Practical Guide to Breast Imaging E-Book
Use of BI-RADS 3 is best validated for round or oval circumscribed solid masses, grouped round or punctate calcifications, and focal asymmetry on a baseline ... 於 books.google.com.tw -
#2.BI-RADS - Wikipedia
BI -RADS is an acronym for Breast Imaging-Reporting and Data System, a quality assurance tool originally designed for use with mammography. 於 en.wikipedia.org -
#3.Practical and illustrated summary of updated BI-RADS for ...
The American College of Radiology (ACR) fifth edition of the Breast Imaging-Reporting and Data. System [BI-RADS] Atlas [1] provides ... 於 www.e-ultrasonography.org -
#4.BI-RADS Category 5 Assessments at Diagnostic Breast ...
Rationale and Objectives The Breast Imaging-Reporting and Data System (BI-RADS) atlas defines category 5 assessments as appropriate only for lesions that ... 於 tmu.pure.elsevier.com -
#5.bi-rads中文
為了要讓檢查結果報告有其一致性,利用了美國放射醫學學會所發展的系統:乳房影像報告與資料系統(BI-RADS),其英文全名是Breast Imaging Reporting and Data System, ... 於 www.omarwraikat.me -
#6.BI-RADS-Net: An Explainable Multitask Learning Approach for ...
This paper introduces BI-RADS-Net, a novel explainable deep learning approach for cancer detection in breast ultrasound images. 於 arxiv.org -
#7.乳房攝影及BIRADS診斷| 醫學影像學習園地 - 中國醫藥大學
乳房攝影及BIRADS診斷 Mammogram-ACR BIRADS. 文/ 夏顥瑜 陳昱廷 廖庭儀 鄭雯欣 李偉慈 李瑋涓 楊有容 陳彥佑. 簡介 乳房X光攝影能偵測乳房微鈣化的轉變及早期病變的不 ... 於 www2.cmu.edu.tw -
#8.Classification BI-RADS - - Depistagesein.ca
La classification BI-RADS (Breast Imaging-Reporting And Data System) est utilisée par les radiologistes lors de la mammographie, de l'échographie et de ... 於 www.depistagesein.ca -
#9.BI-RADS Classification for Management of Abnormal ...
(J Am Board Fam Med 2006;19:161–4.) The Breast Imaging Reporting and Data System. (BI-RADS) was developed in 1993 by the American. College of Radiology ... 於 www.jabfm.org -
#10.MRI in the Assessment of BI-RADS® 4 lesions - LWW
The American College of Radiology (ACR) Breast Imaging-Reporting and Data System (BI-RADS) lexicon, 於 journals.lww.com -
#11.乳房超音波影像之自動BI-RADS分級與電腦輔助診斷
乳房影像報告暨資料分析系統(BI-RADS)針對超音波影像的腫塊組織定義五種語彙用於評定BI-RADS等級以評估腫瘤的惡性程度。於是,我們提出一個自動BI-RADS分級系統用於 ... 於 www.airitilibrary.com -
#12.Journal of Surgical Ultrasound
Purpose: The malignancy rates within the Breast Imaging Reporting and Data System (BI-RADS) category 4a and 4b lesions were examined, and the correlations with ... 於 www.jsu.or.kr -
#13.腫瘤亦有「審美標準」?認識BIRADS評分|曾頤欣醫生
有不少病人收到乳房造影和超聲波片後,發現當中有一個名為「BIRADS」的評分,對此皆充滿疑惑,今日,就讓我向大家解惑。 良. 於 medicalinspire.com -
#14.乳腺影像报告数据系统(BI-RADS) - MedSci-梅斯医学
乳腺影像报告数据系统(BI-RADS). 分级, 描述. 0类, 需要其他影像检查进一步评估或与前片比较。 於 m.medsci.cn -
#15.BI-RADS® fifth edition: A summary of changes - ScienceDirect
The Breast Imaging Reporting and Data System (BI-RADS® ) is a standardized system of reporting breast pathology as seen on mammogram, ultrasound, ... 於 www.sciencedirect.com -
#16.2018.11.27 [醫影] 乳房影像BI-RADS - 醫學記事
BI -RADS * BI-RADS ® (Breast Imaging Reporting and Data System 乳房影像報告與資料系統) 是美國放射學會ACR(American College of Radiology )為了 ... 於 adamrun7.blogspot.com -
#17.ACR BI-RADS Atlas - Amazon.com
The 5th Edition of the BI-RADS Atlas provides standardized breast imaging findings terminology, assessment structure, report organization, ... 於 www.amazon.com -
#18.Breast imaging-reporting and data system (BI-RADS)
BI -RADS (Breast Imaging-Reporting and Data System) is a risk assessment and quality assurance tool developed by American College of Radiology that provides ... 於 radiopaedia.org -
#19.乳房健康與乳癌知識衛教網
為了要讓檢查結果報告有其一致性,利用了美國放射醫學學會所發展的系統:乳房影像報告與資料系統(BI-RADS),其英文全名是Breast Imaging Reporting and Data System, ... 於 www.mdesign.tw -
#20.ACR BI-RADS®-Atlas der Mammadiagnostik | SpringerLink
BI -RADS®: Bildgebende Mammadiagnostik mit Mammografie, Ultraschall und MR! Dieses Buch ist die offiziell genehmigte, deutsche Übersetzung des ACR ... 於 link.springer.com -
#21.New Clinical Rule Helps Distinguish Between BI-RADS 3 and ...
Women who have indeterminate BI-RADS© 3 or BI-RADS© 4 breast masses should undergo a diagnostic biopsy instead of having follow-up imaging ... 於 www.diagnosticimaging.com -
#22.A Nomogram Combining Radiomics and BI-RADS - Nature
Predicting Breast Cancer in Breast Imaging Reporting and Data System (BI-RADS) Ultrasound Category 4 or 5 Lesions: A Nomogram Combining ... 於 www.nature.com -
#23.Dr. Breast專欄: 【乳房攝影報告Q&A】
乳房攝影報告看不懂,"BI-RADS 0 "是什麼? ... 乳房攝影結果BIRADS分類:美國放射線學會將乳房攝影結果訂為六個分級,這個分級系統被稱為乳房影. 於 www.nbic.com.tw -
#24.BI-RADS - Breast Imaging Reporting and Data System - SciELO
Breast Imaging Reporting and Data System - BI-RADS®: positive predictive value of categories 3, 4 and 5. A systematic literature review. 於 www.scielo.br -
#25.Breast Diseases: An Evidence-Based Pocket Guide
BI -RADS® category 1: no relevant findings. Annual mammography is recommended. • BI-RADS® category 2: benign findings – masses containing radiolucent area ... 於 books.google.com.tw -
#26.Clinical Outcome of Breast Cancer BI-RADS 4 Lesions During ...
A total of 392 cases of BI-RADS 4 were identified and 320 could be sub-categorised as 4a, 4b and 4c. Overall malignant positive results were ... 於 www.koreascience.or.kr -
#27.BI-RADS 4 breast lesions: could multi-mode ultrasound be ...
Therefore, the differential diagnosis of benign and malignant BI-RADS 4 breast lesions has become extremely important. Thus, in this paper, we investigated the ... 於 gs.amegroups.com -
#28.Utilization and Cancer Yield of BI-RADS 3 Lesions Detected ...
Utilization and Cancer Yield of BI-RADS 3 Lesions Detected on High Risk Screening Breast MRI. Results to be exhibited at ARRS 2019 Annual Meeting. 於 arrs.org -
#29.乳房X光影像BI-RADS分類與嚴重程度排序 - FIND
醫師評估乳房病變嚴重程度是參考乳房成像報告與數據系統(Breast Imaging-Reporting and Data System,BI-RADS)內制定項目,該些項目包括:乳房病變的 ... 於 www.find.org.tw -
#30.(PDF) Comparing BI-RADS 2013 With the 2003 Edition: Has It ...
PDF | On May 31, 2019, Afsaneh Alikhassi published Comparing BI-RADS 2013 With the 2003 Edition: Has It Worked Better? 於 www.researchgate.net -
#31.Mammogram Reports and BI-RADS: Category 2 - Diagnostic ...
So, on to BIRADS 2 – Benign findings. A BI-RADS category 2 at the end of your report means that the mammogram, breast ultrasound and/or MRI ... 於 www.dic-kc.com -
#32.Benign (BI-RADS 2) lesions in breast MRI - Clinical Radiology
The frequency of malignancy in breast lesions classified as BI-RADS® 2 is zero. •. Breast biopsies are unnecessary in these cases. Aim. To ... 於 www.clinicalradiologyonline.net -
#33.女性常用乳房腫瘤檢查(上) - 台北醫學大學附設醫院
女性,由於乳腺比較緻密,比較不適合利用乳房攝影| BI-RADS 0:影像屬性不明,需安排進一步的影像. 來偵測早期乳癌;但是對有乳癌家族遺傳史的女性,. 於 www.tmuh.org.tw -
#34.Understanding BI-RADS | Barnes-Jewish St. Peters Hospital
BI -RADS is an acronym for Breast-Imaging Reporting and Data System that represents a standardized reporting system for breast imaging published by the ... 於 www.bjsph.org -
#35.What BIRADS on your mammography mean? | CK Birla Hospital
Breast Imaging Reporting and Data System (BIRADS) is a standardized system used by radiologists to enable uniformity in assessing ... 於 www.ckbhospital.com -
#36.Assessment and Management of Challenging BI-RADS ...
Challenging BI-RADS Category 3. Mammographic Lesions1. Breast Imaging Reporting and Data System (BI-RADS) category. 3 lesions are probably benign by ... 於 portaldeboaspraticas.iff.fiocruz.br -
#37.乳房攝影報告格式推廣(2020.12.22 更新) - 中華民國放射線醫學會
為了增進國內乳攝篩檢報告的一致性,以下資料提供參考,包含: (1) 附件一、BI-RADS 5th Location of Lesion (106.3.14公告). (2) 附件二、乳房攝影影像報告應有的內容 ... 於 www.rsroc.org.tw -
#38.Breast Imaging Reporting and Database System (BI-RADS)
Radiologists in the United States and some other countries use the Breast Imaging Reporting and Database System, or BI-RADS, to report the ... 於 www.breastcancer.org -
#39.World Congress of Medical Physics and Biomedical Engineering ...
The correlation between computerised results with BI-RADS classification and ... Keywords— Computerised assessment, breast density, BIRADS classification, ... 於 books.google.com.tw -
#40.Clinical impact of BI-RADS classification in Taiwanese breast ...
On univariate analysis, the mammographic findings (BI-RADS 5 versus BI-RADS 0–4) were statistically significantly associated with five-year overall survival and ... 於 www.ejradiology.com -
#41.乳房檢查報告單上的BI-RADS 分級是什麼意思? - 每日頭條
BI -RADS 即Breast imaging reporting and data system,美國放射協會建立了「乳腺影像報告和數據系統」。目前被國際上廣泛採用,使乳腺病變的檢查報告 ... 於 kknews.cc -
#42.Predictive performance of BI-RADS magnetic resonance ...
category 4 lesions, as described in the Breast Imaging Reporting and Data System (BI-RADS®) lexicon, as well as to test the predictive. 於 webcir.org -
#43.Issues in Diagnostics and Imaging: 2013 Edition
On univariate analysis, the mammographic findings (BI-RADS 5 versus BIRADS 0-4) were statistically significantly associated with five-year overall survival ... 於 books.google.com.tw -
#44.Application of MRI Radiomics-Based Machine Learning Model ...
Materials and Methods: A total of 178 contralateral BI-RADS 4 lesions (97 malignant and 81 benign) collected from 178 breast cancer patients ... 於 www.frontiersin.org -
#45.A Practical Guide to Understanding BIRADS - DocPanel
BIRADS is a numerical scoring system used to evaluate the risk of breast cancer based on a mammogram, breast ultrasound, or breast magnetic ... 於 www.docpanel.com -
#46.BI-RADS Update
BI -RADS Update. Cecilia L. Mercado, MD. INTRODUCTION. The American College of Radiology (ACR) Breast. Imaging Reporting and Data System (BI-RADS). 於 incan-mexico.org -
#47.Guide to Mammography Reports: BI-RADS Terminology
Mammography reports use specific terminology from the Breast Imaging Reporting and Data System (BI-RADS) to describe findings and to assign ... 於 www.aafp.org -
#48.Follow-up 6 Months After BI-RADS 3 Findings on ...
Of the total population of women given BI-RADS 3 assessment after screening mammography recall, 810 (1.9%) were diagnosed with cancer. More, ... 於 www.cancernetwork.com -
#49.BI-RADS Scoring System for Breast Imaging and Mammograms
The BI-RADS scoring system is a numerical scale used in breast imaging and mammogram reports to help detect breast cancer. 於 www.verywellhealth.com -
#50.What to Know About Your BI-RADS Score - WebMD
BI -RADS stands for Breast Imaging Reporting and Data System. It is an assessment tool used to rate the results of a mammogram test. 於 www.webmd.com -
#51.The application of BI-RADS feature in the ultrasound breast ...
This paper proposed a breast tumor computer aided diagnosis (CAD) system utilized the Breast Imaging Reporting and Data System (BI-RADS) features. 於 ieeexplore.ieee.org -
#52.Inappropriate use of BI-RADS Category 3: Learning from ...
BI -RADS 3 is strongly discouraged as a final assessment from a screening mammogram. Finally, BI-RADS 3 is not to be used as a category of ... 於 www.appliedradiology.com -
#53.乳房攝影的解讀系統,BI-RADS - 中華民國婦癌基金會
乳房攝影的解讀系統,BI-RADS · 1:正常。 · 2:良性發現。 · 3:可能為良性發現。 · 4:懷疑異常。 · 5:高度懷疑為惡性腫瘤。 · 6:切片已證實為惡性腫瘤。 於 www.femalecancer.org.tw -
#54.乳腺超声BI-RADS分类及处理建议 - 知乎专栏
BI -RADS 0:此类主要是指利用超声检查可能无法确诊,而需进行其他的影像学检查诊断,进一步评估或与前片比较。 BI-RADS 1:阴性,无异常发现。 於 zhuanlan.zhihu.com -
#55.乳癌篩檢、確診與治療處置流程 - 長庚醫院
乳房X光攝影篩檢. 陰性. 陽性. BIRADS 0. BIRADS 3. BIRADS 1/2. BIRADS 4/5. 定期檢查. 短期追踪. 複檢. 組織生檢. BIRADS 4/5. BIRADS 1/2/3. 嘉義長庚紀念醫院 ... 於 www1.cgmh.org.tw -
#57.Results of Short-Term Follow-Up in BI-RADS 3 and 4a Breast
Information on clinical history, lesion characteristics on ultrasound (US), mammography, and magnetic resonance imaging (MRI), BI-RADS ... 於 www.karger.com -
#58.Breast Imaging Reporting and Data System (BI-RADS)
BI -RADS terms most frequently used are described, and most are illustrated by figures. In addition, the rules for a facility and radiologist audit are ... 於 oxfordmedicine.com -
#59.Power Doppler and gray-scale sonography standardized by BI ...
Power Doppler and gray-scale sonography standardized by BI-RADS for the differentiation of benign postoperative lesion and local recurrence ... 於 www.spandidos-publications.com -
#60.超声造影对不同大小BI-RADS 4类乳腺结节的良恶性定性诊断研究
收集2017年10月至2019年5月上海交通大学附属第一人民医院的467例患者506个常规超声定性为BI-RADS 4类乳腺结节,分为大结节组(最大径线>20 mm,198个)和小 ... 於 rs.yiigle.com -
#61.Clinical Application of the BI-RADS Final Assessment to ...
OBJECTIVE. The objective of our study was to report the results of classification of sonographic findings according to BI-RADS and to calculate the positive ... 於 www.ajronline.org -
#62.哪些是乳癌症狀的危險因子 - 亞東醫院
* 若懷疑為惡性腫瘤(BI-RADS 4或5),則會建議接受穿刺或切片取得細胞以確立診斷。 目前政府提供的免費乳房X光攝影檢查條件 * 45-69歲婦女,每2年1次 * 40-44 ... 於 www.femh.org.tw -
#63.BIRADS, Breast Ultrasound, mamography - SlideShare
BIRADS, Breast Ultrasound, mamography. 1. Dr. Mohit Goel JRIII 27/2/15; 2. BI-RADS® is designed to standardize breast imaging reporting and ... 於 www.slideshare.net -
#64.What are the BI-RADS assessment categories based on ...
BI -RADS assessment categories can be summarized as follows: Category 0 - Need additional imaging evaluation Category 1 - Negative Category 2 - Benign ... 於 www.medscape.com -
#65.Performance of machine learning software to classify breast ...
We extracted the main ten radiomic features based on the BI-RADS lexicon and classified the lesions as benign or malignant using a bottom-up ... 於 eurradiolexp.springeropen.com -
#66.ACR BI-RADS Atlas 5th Edition (Book) - 力大圖書
The 5th Edition of the BI-RADS® Atlas provides standardized breast imaging findings terminology, assessment structure, report organization, and a classification ... 於 www.leaderbook.com.tw -
#67.BIRADS 2 3 4 and 5: What does it mean? - Breast Cancer
BIRADS or 'BI-RADS stands for Breast Imaging Reporting and Data System and was established by the American College of Radiology. 於 breast-cancer.ca -
#68.乳房檢查報告的判讀- 簡介BIRADS
BIRADS (The Breast Imaging Reporting and Data System)。以下就乳房X光攝影. 和乳房超音波檢查分別介紹:. 一、乳房X光攝影. 乳房X光攝影檢查依乳房組織的密. 於 www.tafm.org.tw -
#69.知多啲:X光造影年輕女性未必合用 - 明報健康網
【明報專訊】乳房影像檢查報告,有BI-RADS(Breast Imaging Reporting and Data System)分級,判斷患乳癌的機率。BI-RADS是美國放射學會(American ... 於 health.mingpao.com -
#70.BI-RADS, C-RADS, CAD-RADS, LI-RADS, Lung-RADS, NI ...
The prototype system Breast Imaging Reporting and Data System (BI-RADS) was first published by the ACR in 1993 to address the lack of ... 於 pubs.rsna.org -
#71.禾馨民權婦幼診所- 如何了解乳房影像判讀書面報告
美國放射科醫學會(ACR)公佈一套乳房影像報告系统(BI-RADS),將乳房X光攝影、乳房超音波、乳房磁振造影(MRI)的檢查結果標準化,建立起醫師間及醫師與受檢者間的 ... 於 www.dianthus.com.tw -
#72.Comparing BI-RADS 5th Edition Descriptors with the BI-RADS ...
The Breast Imaging Reporting and Data System (BI-RADS) has standardized and facilitated the description and management of microcalcifications identified on ... 於 sites.kowsarpub.com -
#73.Bi-RADS for Mammography and Ultrasound 2013 - The ...
BI -RADS® is designed to standardize breast imaging reporting and to reduce confusion in breast imaging interpretations. It also facilitates ... 於 radiologyassistant.nl -
#74.乳腺X线摄影检查报告单上显示“BI-RADS:1、2 - 医学微视
(采访)如果在乳腺X线摄影报告单上面看见这个“BI-RADS:1、2、3”这些数字是指的什么呢?这个BI-RADS一般会写在结论的这个部分,像肿块、钙化、结构扭曲都应该在前面 ... 於 www.mvyxws.com -
#75.CBR apresenta BI-RADS - Colégio Brasileiro de Radiologia
CBR Introduces BI-RADS® ... Imaging professionals will have access to an important tool for their routine: the Brazilian College of Radiology and Diagnostic ... 於 cbr.org.br -
#76.SBI Webinars: Introduction to BI-RADS - Society of Breast ...
This talk will present the history and rationale behind the development of BI-RADS, proper use of lexicon terms for mammography, ultrasound, and MRI and proper ... 於 www.sbi-online.org -
#77.Breast Imaging Reporting and Data System - StatPearls - NCBI
Breast imaging-reporting and data system (BI-RADS) is a classification system proposed by the American College of Radiology (ACR) in 1986 ... 於 www.ncbi.nlm.nih.gov -
#78.Definition of BI-RADS - NCI Dictionary of Cancer Terms
BI -RADS ... A method used by radiologists to interpret and report in a standardized manner the results of mammography, ultrasound, and MRI used in breast cancer ... 於 www.cancer.gov -
#79.BI-RADS® fifth edition: A summary of changes - MD Anderson ...
The Breast Imaging Reporting and Data System (BI-RADS® ) is a standardized system of reporting breast pathology as seen on mammogram, ultrasound, ... 於 mdanderson.elsevierpure.com -
#80.ACR BI-RADS® Atlas 5th Edition - American College of ...
BI -RADS is a comprehensive guide providing standardized breast imaging terminology, report organization, assessment structure and a classification system ... 於 www.acr.org -
#81.乳篩結果BI-RADS 0或4 快回醫院複檢| 乳癌| 癌症防治
... 部國健署提供45歲以上女性每2年一次免費篩檢,若篩檢結果為BI-RADS 0和BI-RADS 4代表疑似乳癌個案,就會通知民眾前往複檢,但仍有2成患者未複檢。 於 health.udn.com -
#82.BI-RADS Score: Understanding Your Mammogram Results
The BI-RADS score is an acronym for the Breast Imaging Reporting and Database System score. It's a scoring system radiologists use to describe mammogram ... 於 www.healthline.com -
#83.BI-RADS density categorization using deep neural networks
The Breast Imaging and Reporting Data System (BI-RADS) density score is a qualitative measure and thus subject to inter- and ... 於 www.spiedigitallibrary.org -
#84.Dense breast tissue: What it means to have ... - Mayo Clinic
Levels of density are described using a results reporting system called Breast Imaging Reporting and Data System (BI-RADS). The levels of density are often ... 於 www.mayoclinic.org -
#85.組織生檢短期追蹤複檢定期檢查
Surveillance Imaging (BI-RADS Category 3) Algorithm. 文件版本: 第一版 ... 診斷式乳房攝影(局部放大,特殊攝影). BIRADS 2. BIRADS 3. BIRADS 4/5. 於 org.vghks.gov.tw -
#86.MR BI-RADS Lexicon and Usage - STATdx
Abbreviations. Breast Imaging Reporting and Data System (BI-RADS) for Magnetic Resonance (MR) Imaging. Terms may undergo revision; consult current edition of ... 於 app.statdx.com -
#87.何謂乳房密度?
即使您的乳房密度高,您. 也不應該驚慌,但就應該找醫護人員談談您罹. 患乳癌的整體風險。 類別. 說明. BI-RADS A. 您的乳房幾乎全部由非密集(脂肪). 於 www.bccancer.bc.ca -
#88.Application of ultrasound artificial intelligence in the ...
The classification of Breast Imaging Reporting and Data System 4A (BI-RADS 4A) lesions is mostly based on the personal experience of doctors ... 於 bmccancer.biomedcentral.com -
#89.Is “Probably Benign” Good Enough for BI-RADS 3 Patients?
For these women and other women with a BI-RADS 3 score, an additional diagnostic tool that provides accurate information on their breast cancer ... 於 www.provistadx.com -
#90.Comparing Visually Assessed BI-RADS Breast Density and ...
Based on the BI-RADS classification, 40.8% of the women had 'heterogeneously or extremely dense' breasts. The median volumetric percent ... 於 journals.plos.org -
#91.乳腺超声报告中的BI-RADS,表示什么意思?_检查 - 手机搜狐网
乳腺BI-RADS(Breast Imaging Reporting And Data System)分类是美国放射学会(ACR)制定并推荐的“乳腺影像报告和数据系统”,是乳腺影像学检查分类 ... 於 www.sohu.com -
#92.當年度經費: 308 千元 - 政府研究資訊系統GRB
在臨床診斷中,醫師對於超音波影像中的可疑物件利用定義ACR BI-RADS 的描述項目描述特徵,並藉此估計惡意的可能性,在本研究計畫中,將針對描述特徵的所有特徵,包含 ... 於 www.grb.gov.tw -
#93.Routine screening for BI-RADS lesions on auto | EurekAlert!
Return to routine screening for BI-RADS 3 lesions on supplemental automated whole-breast ultrasound substantially reduces the recall rate, ... 於 www.eurekalert.org -
#94.Understanding Your Mammogram Report - American Cancer ...
What is a BI-RADS assessment category? ... Doctors use a standard system to describe mammogram findings and results. This system (called the ... 於 www.cancer.org -
#95.台灣民眾, 乳房攝影在未經進一步查證, 即判定陰性(BiRADs 1 ...
在國際上乳房攝影 (BiRADs) 的判讀, 分為 0,1,2,3,4,5. 其中 1 為確認陰性(negative), 2 為確認良性 (benign), 3,4,5 則為不同程度的疑似惡性 (maliganant). 其中, BiRADs ... 於 blog.xuite.net -
#96.A New Computer-Aided Diagnosis System with Modified ...
The language used to describe abnormalities in mammographic reports is based on the Breast Imaging Reporting and Data System (BI-RADS). 於 www.hindawi.com -
#97.BI-RADS 3 on Screening Breast Ultrasound: What Is It and ...
A probably, benign Breast Imaging Reporting and Database System (BI-RADS) 3 (1), assessment was first codified for mammographic findings proven ... 於 academic.oup.com